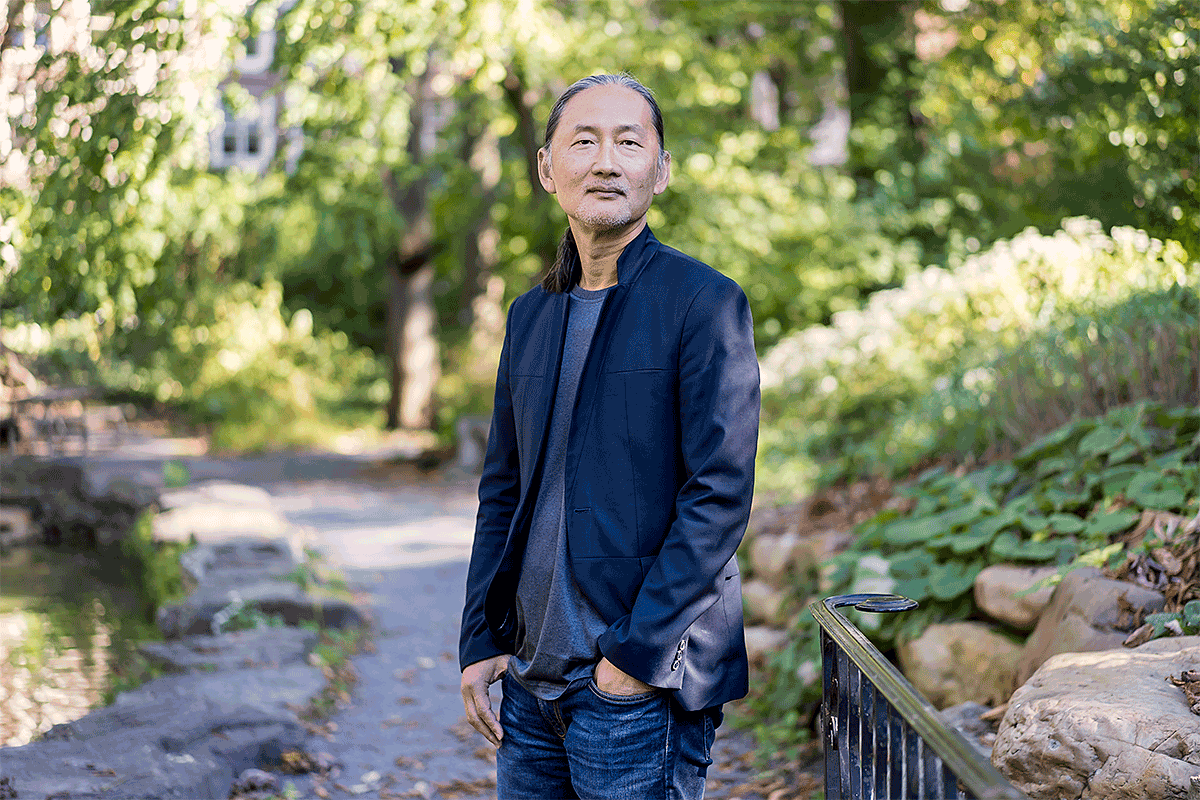
Junhyong Kim, Patricia M. Williams Term Professor of Biology
Photo by me
Tuesday, December 1, 2015
By Blake Cole
Illustrations by Jon Krause
Photo by Shira Yudkoff
Social media sites store hundreds of billions of photos, while super-retailers are clocking in millions of sales per hour. Deep space telescopes and powerful particle accelerators produce vast quantities of information that can transform the state of science—as long as that data can be stored.
How can we retain, process, and make sense of the seemingly endless amount of complex information that society produces? This is the quintessential challenge of big data in our modern technological age—and it will only escalate as our digital footprint grows with time.
“The world is awash in data of all types,” says Junhyong Kim, Patricia M. Williams Term Professor in Biology, previous co-director of the Penn Genomics Institute, and current co-director of the Penn Program in Single Cell Biology. “It affects every discipline, from the sciences to the humanities to even the arts.” Given the volume and complexity of data, computation on a previously unimaginable scale has become a necessity. “Computer processing,” Kim notes, “is involved in every aspect of knowledge-generating activity, including data access, data integration, data provenance, as well as visualization, modeling, and theorizing.”
Kim and his co-investigator Zack Ives, Professor and Markowitz Faculty Fellow in Computer and Information Science in Penn Engineering, are working at the frontiers of the expanding universe of big data with the help of a grant from the National Institutes of Health (NIH), through its BD2K (Big Data to Knowledge) initiative. Their main goal: Design a computer software program that can handle the extensive data management requirements of single-cell genomics and other genome-enabled medicine—and, while they’re at it, to help address big data issues that may have an impact on a range of endeavors within and beyond the research community.
“The really big questions in science can only be answered by collecting volumes of data from multiple sites, putting it together, and doing big data analytics over the results,” says Ives. “Unfortunately right now the data management tools available aren’t really up to the task.”
It turns out Kim’s own field, single cell genomics, offers a perfect laboratory for exploring the problem of marshaling big data. “People understand that there are different types of cells—that brain cells are different from heart cells and so on,” says Kim. “But there’s a tendency to view certain kinds of groups of cells like bricks in the wall, when it turns out that every cell is actually quite different.” To understand the function of individual cells in the human body, Kim’s lab and collaborator James Eberwine, Elmer Holmes Bobst Professor of Pharmacology at the Perelman School of Medicine and co-director of the Penn Program in Single Cell Biology, look at individual cells at a minute level of detail, using groundbreaking technology—technology that produces massive amounts of data.
The process involves multiple steps: isolating ribonucleic acid (RNA) from individual cells, amplifying it so that there are enough molecular copies to process, and, finally, sequencing it in order to reveal gene expressions. This glimpse at the function and behavior of individual cells provides a scientific foundation that may translate to important medical advances, such as the development of pharmaceuticals that target specific genes to treat degenerative diseases like Alzheimer’s. “If you look at your face and see where your freckles appear, it’s clear that there’s a lot of heterogeneity,” says Kim. “So when we get degenerative diseases, it’s not like your whole brain goes at once. Some cells go and some cells don’t. And that’s due to this cell-to-cell variation we are working to understand."
Keeping track of the complex experimental processes and data analysis involved in genomics research is extremely challenging, and this is the problem that the new BD2K-funded project, titled “Approximating and Reasoning about Data Provenance,” is addressing. The technological advances that have brought the cost of sequencing the human genome down from $3 billion to $1,000 have also produced a data deluge, and processing and managing this volume of information is the main bottleneck in research and translational applications.
The technological advances that have brought the cost of sequencing the human genome down from $3 billion to $1,000 have also produced a data deluge, and processing and managing this volume of information is the main bottleneck in research and translational applications.
In order to chart the “provenance” of the data—the “where” and “how” that data came about—each step has to be meticulously traced. “When patients come in for neurosurgery, whether it’s for epileptic surgery or tumor resection, there is a lot of information associated with those patients and why they are there,” says Kim, whose lab studies several types of cells, including human heart cells, immune cells, olfactory sensory cells, and stem cells. “This includes who the patients are, what previous pathologies they had, what kind of medication they were on, and even who the surgeons were.” The variables continue in the lab. Once the tissue comes in, it’s separated into various processing streams. Sometimes, for example, it’s taken directly for examination, while other times a specimen is frozen. Eventually the RNA of individual cells is collected and amplified, a multi-step process involving various reagents. All of these variables must then be processed by the sequencing software—the real hard-drive hog.
The tools and methods used to track data may ultimately determine the weight accorded to the results within the scientific community. “When we want to compare data from different sources, we need to understand whether it was created using the same recipe,” Ives says. “We’re trying to come up with better ways of storing, collecting, and making use of provenance to help scientists.” Any software Kim and his collaborators develop will be open source so researchers around the world can iterate and improve the platform down the road.
The team attacking this problem includes Kim, who has an adjunct appointment in the computer science department, and Ives, along with Susan Davidson, Weiss Professor of Computer and Information Science, and Sampath Kannan, Henry Salvatori Professor and Department Chair in Computer and Information Science. As Kim notes about this cross-pollination of biology with computer science, “I have been interacting with these faculty for over 10 years now, so we share a language. The key to this kind of interdisciplinary research is to be willing to work on something trivial at the beginning. And then that develops into something deep and interesting.”
"The key to this kind of interdisciplinary research is to be willing to work on something trivial at the beginning. And then that develops into something deep and interesting.”
“It’s a very exciting opportunity for me as a computer scientist to work on problems that can have real impact,” says Ives. “Here we not only have an opportunity to come up with new algorithms and new models, but to produce software that people in many different biomedical fields will find useful.”
Kim adds, “This project is an example of the kind of interdisciplinary research that really is important for us all to be able to do across school boundaries. In addition to the Arts and Sciences, this impacts Medicine and Engineering. And that’s sort of the great thing about the University—being able to do that.”
According to Junhyong Kim, Patricia M. Williams Term Professor in Biology and a co-director of the Penn Program in Single Cell Biology, big data isn’t just a challenge for scientists. He argues that students in all fields need to be exposed to technology early in the curriculum. This conviction is also reflected in the Penn Arts and Sciences strategic plan, “Foundations and Frontiers,” which identifies big data as one of eight areas that are key to the School’s future in education as well as research.
Having immersed himself in the challenges associated with big data, Kim has carefully considered its implications for the undergraduate curriculum. “The teachings of the classical liberal arts, for instance, can be used to help non-science and technology students relate to data issues,” he says. He cites the Trivium model from Ancient Greece and the Middle Ages, which encompasses grammar, logic, and rhetoric. “The Trivium can be interpreted in scientific and technological terms. Grammar defines rules, patterns, and objects of interest, which relates closely to how we track and catalog data, as well as methods like machine learning. Logic determines rules of inference and meaning, and can be tied to how we use various data inference models including graphical models of genetic relationships, causal models of disease, and so on. Finally, rhetoric uses grammar and logic to tell a story—or in more scientific terms, a theory. Theory involves not only quantitative modeling but a conceptual framework for guiding the deployment of models—that is, the theater for the scientific story.”
This construct has inspired Kim’s thinking on potential curriculum-building applications for the future. “Technologies and principles related to computing, modeling, and theorizing are essential to 21st-century higher education, and the skills associated with these technologies should be an essential part of every undergraduate experience.”